Artificial intelligence (AI) is rapidly transforming our world, but behind the scenes, a crucial process fuels its development: dataset annotation. This might sound technical, but it’s essentially the art of adding labels and instructions to raw data, making it understandable for machines.
Imagine a child learning colors. You show them a red ball and say “Red.” This labeling process is akin to dataset annotation. We provide the data (the ball) and the corresponding label (red), allowing the AI model to learn and identify similar objects in the future. In this article, ilovephd gives the complete guide about the Automatic Dataset Annotation.
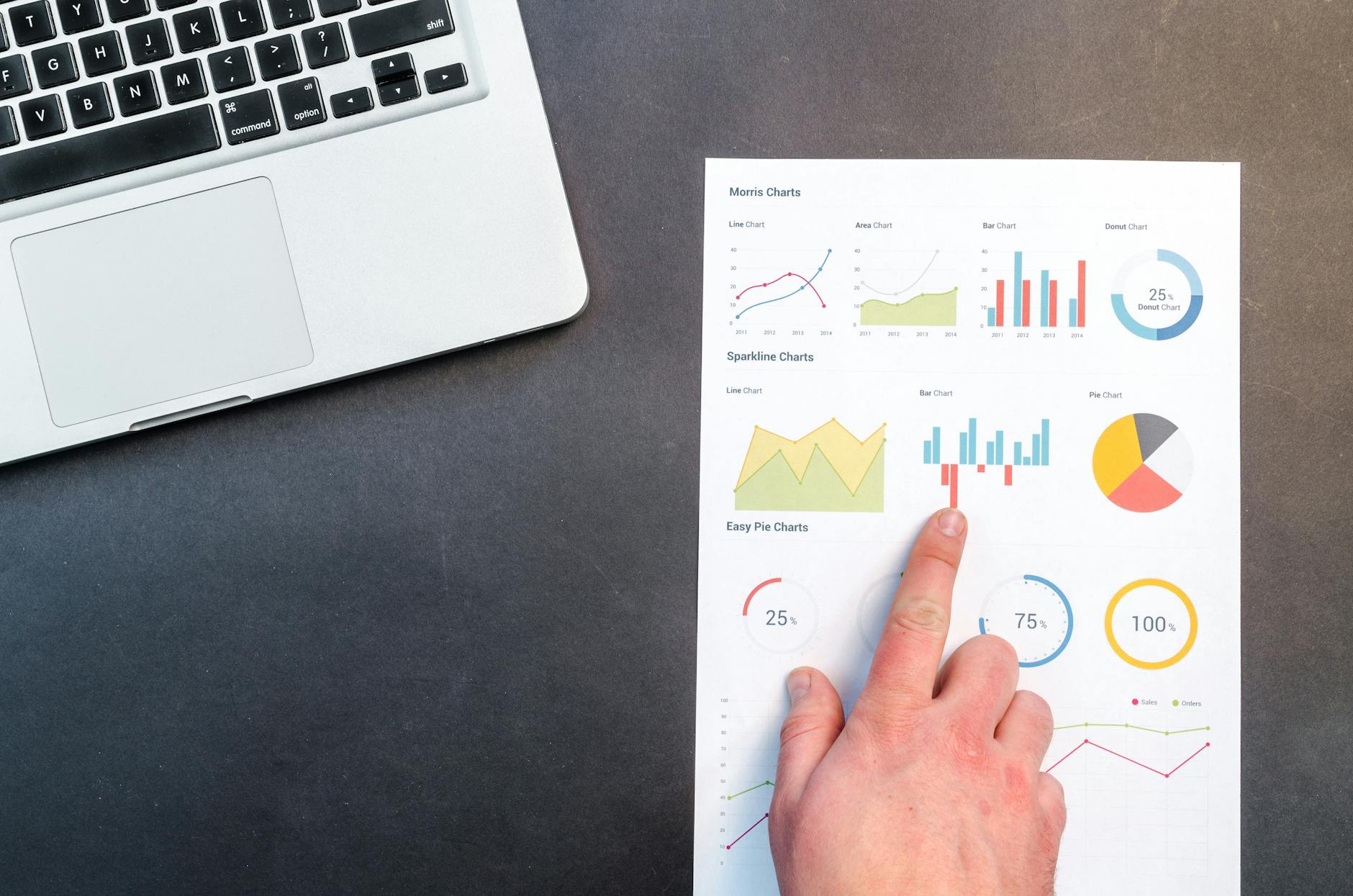
Why is Dataset Annotation Important?
Here’s why dataset annotation is a game-changer for AI:
1. Machine Learning Needs Labels
Machine learning algorithms are like students. They need labeled examples to learn from. Dataset annotation provides these labels, enabling the model to distinguish between a cat and a dog in an image or recognize emotions in speech.
2. High-Quality Data Leads to High-Quality AI
Just like a student performs better with clear instructions, AI models thrive on well-annotated data. Precise labels ensure the model learns the right way, leading to more accurate and reliable AI applications.
3. Different Tasks, Different Annotation Techniques
Data annotation isn’t a one-size-fits-all process. Images might require bounding boxes to identify objects, while text data might involve sentiment analysis or topic classification. The technique depends on the specific AI task.
The Future of Dataset Annotation
As AI continues to evolve, so too will dataset annotation. Here are some exciting trends to watch:
1. Automation and AI-assisted annotation:
While human expertise remains vital, automation tools can streamline repetitive tasks and AI can help identify inconsistencies in annotations.
2. Focus on niche datasets
With increasingly specialized AI applications, the demand for targeted and well-annotated datasets in specific domains like healthcare or finance is on the rise.
3. Collaborative annotation platforms
As the need for large-scale annotation grows, online platforms enabling efficient collaboration among annotators will become even more crucial.
5 Applications of Dataset Annotation
Dataset annotation has a wide range of applications across various industries. Here are five key areas it fuels:
1. Self-Driving Cars
For autonomous vehicles to navigate safely, they need to be trained on massive datasets annotated with information like traffic signs, pedestrians, and lane markings. This allows the car’s AI to identify and react to these objects in real-time.
2. Medical Imaging Analysis
In healthcare, dataset annotation is crucial for training AI models to analyze medical scans like X-rays and MRIs. Annotators label tumors, fractures, and other abnormalities, enabling AI to assist doctors in faster and more accurate diagnoses.
3. Smart Assistants and Chatbots
The ability of virtual assistants and chatbots to understand natural language relies heavily on annotated datasets. Examples include labeling text with sentiment (positive, negative) or intent (request, complaint) to train the AI for effective communication.
4. Content Moderation
Social media platforms and other online services use dataset annotation to train AI for content moderation. Annotators label content as appropriate or inappropriate, hate speech or not, allowing the AI to automatically identify and flag potentially harmful content.
5. E-commerce Product Search
Dataset annotation plays a vital role in enhancing product search on e-commerce platforms. Annotators can label images with product details (clothing size, color) or identify objects in a scene (furniture in a room), allowing the AI to deliver more relevant search results to users.
Top 10 Websites for Dataset Annotation
- Amazon Mechanical Turk (AMT): A popular platform where businesses can outsource microtasks, including data annotation, to a global workforce.
- Labelbox: A user-friendly platform with advanced tools for various annotation tasks, including image, text, and video data.
- CloudFactory: Offers managed annotation services with a global workforce and expertise in complex data types.
- Xylem AI: Specializes in computer vision tasks and provides high-quality data annotation for self-driving cars, robotics, and medical imaging.
- Hivemind: A crowdsourcing platform focused on complex NLP (Natural Language Processing) tasks like sentiment analysis and text summarization.
- Scale AI: Provides high-quality data annotation services with a focus on scalability and security for large datasets.
- Caty.ai: Offers data annotation solutions specifically designed for the needs of the automotive industry.
- Appen: A global leader in data collection and annotation services with a workforce experienced in diverse tasks.
- Lionbridge: Provides data annotation services with a focus on multilingual content, making them ideal for global projects.
- Data Plus Science: Offers data annotation services with expertise in scientific and medical data, catering to research institutions and healthcare companies.
Dataset annotation might seem like a behind-the-scenes process, but it’s the foundation for powerful AI applications that are shaping our future. From self-driving cars to medical diagnosis tools, understanding dataset annotation allows us to appreciate the intricate work that goes into making AI a reality.